5 real-world data product use cases
Examples of how data products are used to solve real-world problems and drive meaningful business impact in B2B SaaS, eCommerce, logistics, and fintech
Data products can be incredibly useful for representing key business and technological processes and how end users use them. If they’re defined well, they represent a specific use case, have clear ownership and priority, and encapsulate logic such as SQL transformations.
As a data practitioner, this means that you can start your day by glancing over the handful of data products that matter to you and confidently know if everything is working as it should – a stark contrast for most compared to looking at a busy Slack channel with dozens of alerts or trying to make sense of hundreds of models and tables.
But getting started with and implementing data products can be complex. And in some cases end up as a theoretical exercise that doesn’t have the desired impact.
Below, we’ll look at five real-world customer stories of companies implementing data products to solve key problems – from monitoring data health to managing ownership and tracking SLAs.
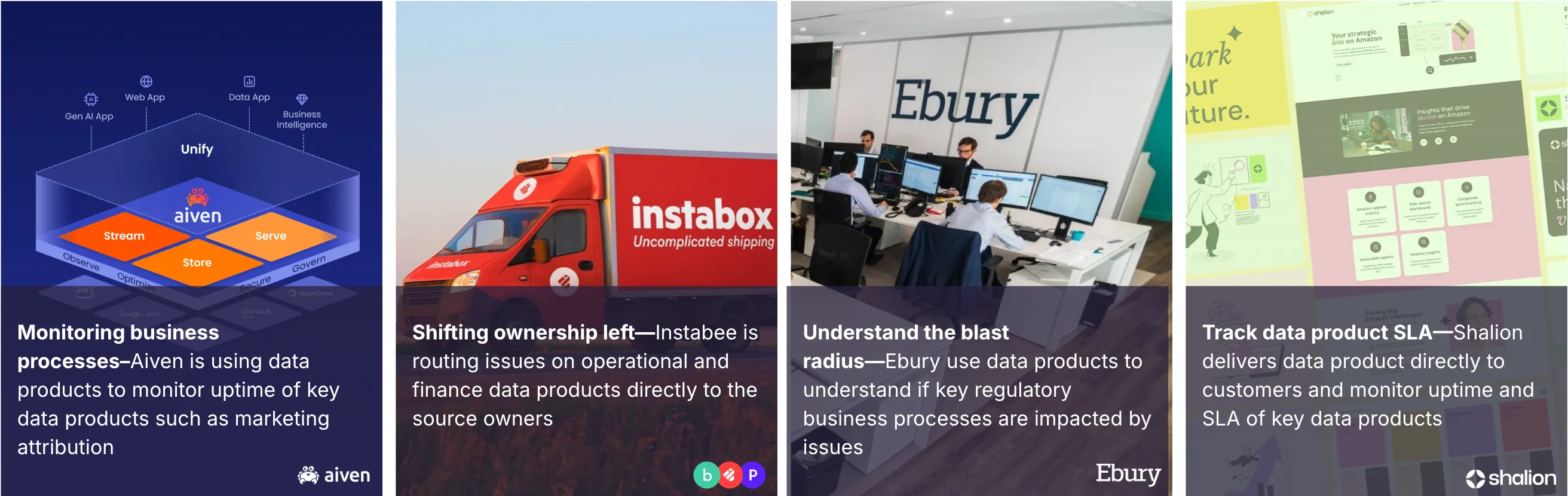
A single pane of glass for monitoring data health
Instabee is taking a technology-first approach to online shipping. Near real-time data is used for key processes and decisions, from operational data such as understanding lead time and volume to forecasting and financial data. If there are issues with this data, stakeholders need to be informed within hours.
Data products have become the lens through which Instabee’s Analytics Engineering team evaluates and reasons about our most important business processes in data. They group data products into areas such as BI and Finance and can instantly see if there are any errors on or upstream of data products.
”The Data Product overview is the first page we open each morning to check if all the nightly runs have run successfully or if there are any errors across dbt and SYNQ anomaly monitors impacting our key data products” – Josefin, Analytics Engineer
By looking at the health of data through the lens of data products and seeing impacted owners, the data team can notify impacted end-users of issues before they start their day.
See Josefin explain how Instabee uses data products for monitoring the health of their most important data
Each data product has a priority ranging from P1 to P3 based on its importance and the team uses that to decide how urgently issues are treated. With this at hand, the data team brings transparency to the business and avoids end-users being the ones to notice issues.
Monitoring business processes instead of models and tables
Aiven takes a data-first approach to decision-making, so timely, accessible, and accurate data is a top priority. The executive leadership team across departments at Aiven relies on data from the data warehouse for weekly business reviews. The data must be accurate for reporting because it impacts decisions made for the business
As part of delivering reliable data to the organization, the team built data products to encapsulate the business’s deliverables, such as sales, marketing, and ARR. In SYNQ, owners of data products can immediately see if there are any issues on the data products or upstream of them and if any ongoing incidents impact the data product. This gives everyone a pane of glass into the uptime of data that can be communicated to the business.
Hear how Aiven groups and segments their data products to be able to capture nuanced use cases
The team got some learnings about what makes useful data products. They started with high-level products such as Sales and Marketing but realized they needed to go a step deeper to have the most impact. For example, if the Marketing data product has an issue, that may be fine. However, if the Attribution data product within Marketing has an issue, they must immediately jump on it. This is the level of detail the data products need to be able to capture for them to be useful.
Confidently assessing blast radius of issues across 10,000 tables
Ebury, a global fintech company specializing in cross-border trade with offices across 29 markets, manages a sprawling data ecosystem. Data is key for central business processes such as models to assess customer risk and reports delivered to regulators. Their stack includes nearly 9,000 BigQuery tables, over 6,000 dbt models, and more than 5,500 dbt tests, supported by hundreds of Looker dashboards.
Before using data products, it was nearly impossible to assess the impact of issues as there were often thousands of tables, dashboards, and dbt models downstream of an issue.
“For model validation, we need to escalate issues to the risk committee before they impact decisions. Not knowing whether our data is reliable isn’t an option. This also applies to regulatory reporting” – Prado Morón, Head of Model Risk Governance.
Data products are grouped into domains such as Credit Risk and Treasury. This overview gives responsible domain owners across the data and business teams all the details they need to know without keeping the context of thousands of irrelevant tables and models in their heads.
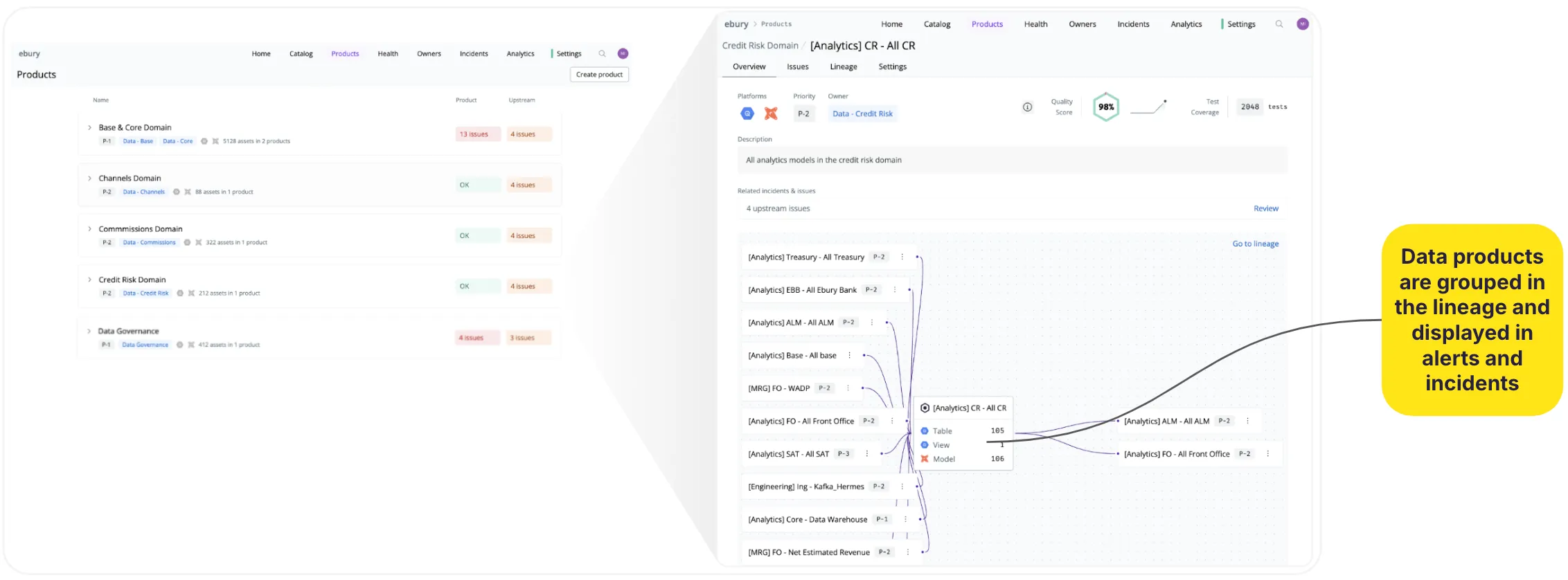
The data team now looks at the health of data through the lens of a few dozen data products and can immediately see if there are any issues and who’s impacted. Before using data products, this was close to impossible as they had to manage and understand the state and dependencies across many thousands of tables.
Shifting ownership to the finance and operations team
A big problem for Instabee was that many issues were not caused by the data team – and in most cases should be resolved directly in source systems. Examples of this include finance data that’s just passed through dbt with few transformations and spreadsheet imports for operational teams.
Instabee created dozens of data products and closely tied them to ownership. The Analytics Engineering team is notified of issues on core models in the data warehouse. But ownership isn’t limited to just the data team. The finance team is notified in the #finance-data-quality-monitoring
Slack channel if there are issues with finance data products. They’ve also extended it to operational use cases where key models rely on manual input data from spreadsheets for fuel data – input issues on these spreadsheets trigger not_null
or unique
dbt test errors and are routed directly to the operations manager responsible for the spreadsheets.
Instabee uses data products to shift ownership to the finance and operations teams
Tracking SLA and uptime for customer-facing data products
Shalion is a global leader in eCommerce intelligence, working with brands such as Heineken, JDE, and Danone. Shalion’s platform unifies digital shelf and retail media insights, helping businesses make data-driven decisions. The solutions deliver product performance data from over 1,000 retailers across over 85 countries, offering full visibility into every aspect of the eCommerce performance.
“Data is our product. Our customers make decisions every day based on the insights we provide. The worst that can happen is if we deliver incorrect data to customers without us being the first to notice” – Alejo, Chief Data Officer
The team is obsessed with being able to monitor the quality of their data products – from the ability to extract source data accurately to the accuracy of the data processing steps. To achieve this, Shalion is breaking down the monitoring of the data quality of data products as follows
- Completeness – has all the data been extracted from retailers on a given day
- Processing performance – what’s the quality of our downstream processes? For example, out of 100 scraped products, what % can our classifier assign a category to
- Accuracy – how well are the data products performing? For example, what is the share of brands reported by Shalion in terms of ads and listings broken down by countries
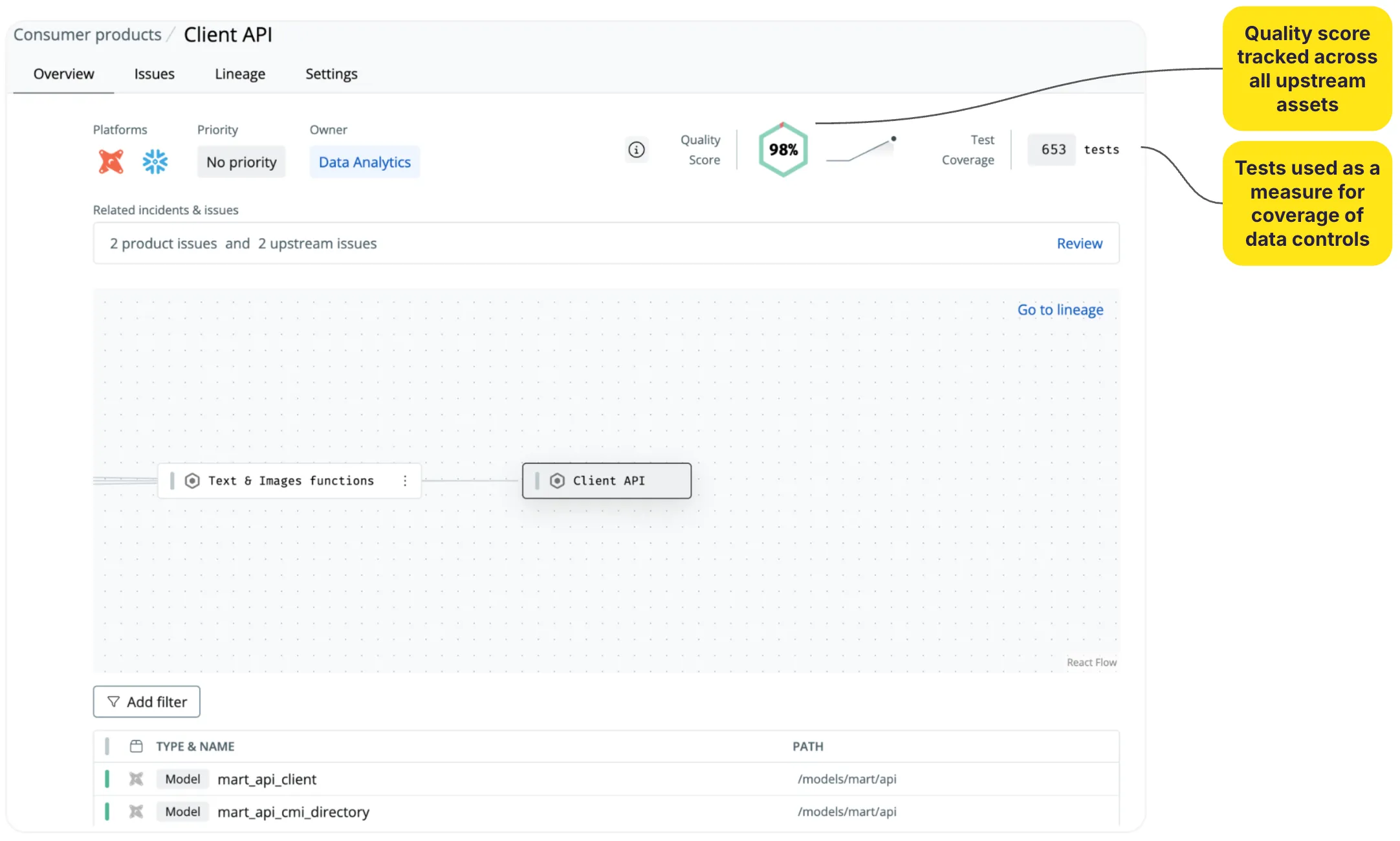
“Next on my list is being able to deliver and commit to SLAs for data products towards our partners. The strength of our data quality combined with our ability to monitor it, will be a competitive advantage for us as a company” – Alejo, Chief Data Officer
Want to learn more about how to get started with data products? Read our guide: The definitive guide to building data products.
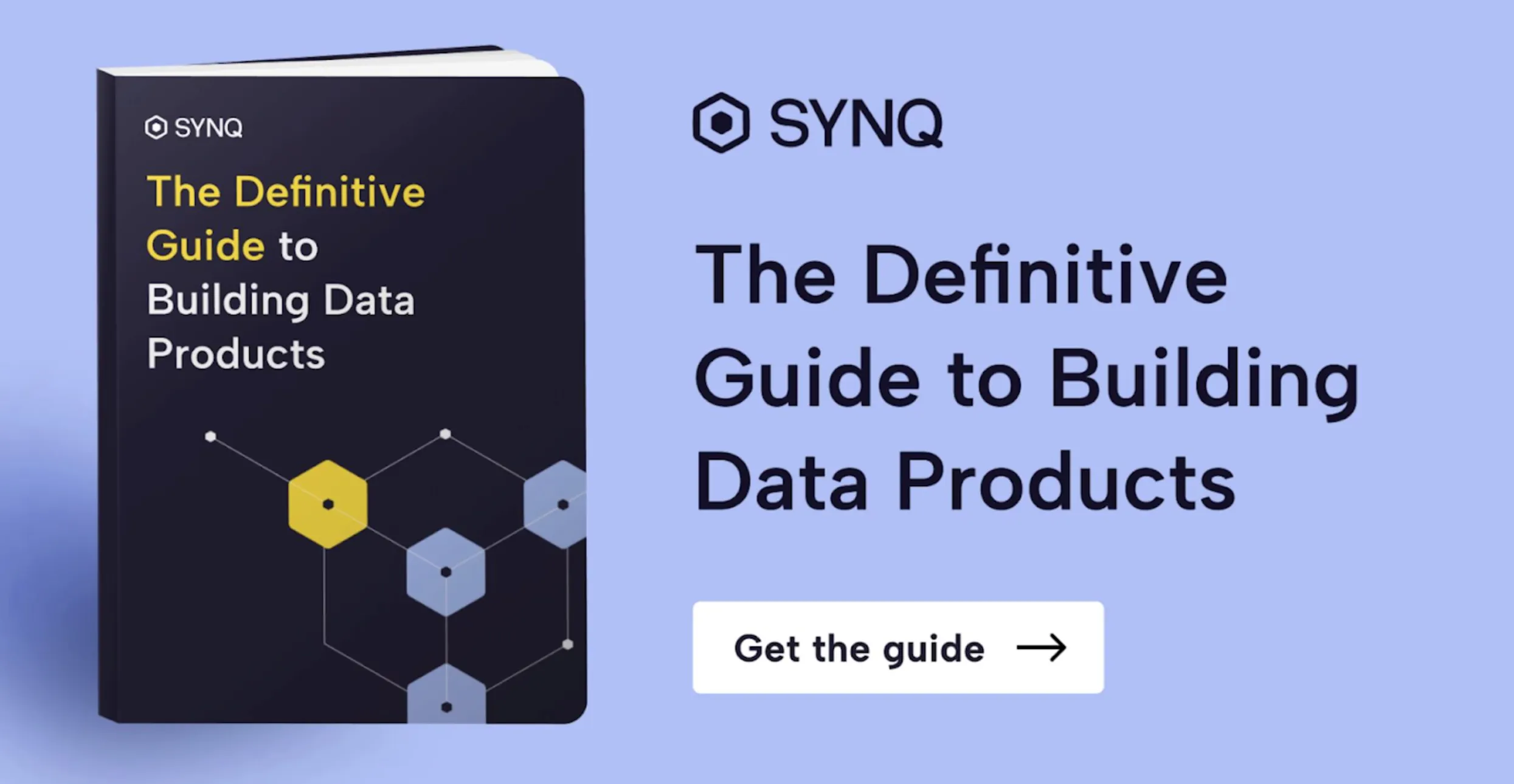
Build with data you can depend on
Join the data teams delivering business-critical impact with SYNQ.